- AI Historical Background
- So What Is AI?
- Confusing terminology
- Myth #1: AI Will Replace Humans
- Myth #2: AI Will Harm Patients
- Myth #3: AI Can Do What Doctors Do
- Myth #4: Better Results with More Data
- Final Thoughts
Table of Contents:
With the advent of computing and digital technology, we can witness the rapid advancement and adoption of cognitive applications. Each wave of development seems more significant than the previous, bringing humanity forward to a breakthrough. The hype around AI is phenomenal; don't get us wrong, we are impressed, but at the same time, we want to be grounded in reality.
Experts compare AI to the invention of the Internet, but how real are its applications, especially in medicine?
Some industry experts aren't very optimistic. Nigam Shah, Chief Data Scientist for Stanford Health Care and Professor of Medicine at Stanford University, warns that only 5% to 10% of all AI applications today can count as valuable.
Defining AI is complex because of the way people use it. A term that describes people's perspective on it is the "AI effect." If there's a skill that artificial intelligence hasn't yet mastered, it's considered a marker of intelligence, but when AI masters that skill, it isn't viewed as such.
Experts believe that one day, there will be artificial general intelligence (AGI) or artificial superintelligence, which is considered to reach human intelligence, be self-aware, and carry out tasks as humans do. But for now, AI is a relatively new concept.
AI Historical BackgroundI
The field of AI was officially founded in the 1950s during the Dartmouth Summer Research Project on Artificial Intelligence. The workshop aimed to determine the human thought process and whether it could be simulated with a machine. At that time, Alan Turing introduced the concept of simulating intelligent behavior and the famous Turing Test to assess if machines can exhibit human intelligence-like behavior.
In medicine, intelligent computer systems have been utilized since the 1970s. For example, CASNET combined causal explanations of glaucoma with empirical knowledge to help guide patient management. Since then, AI has significantly developed in multiple fields, such as robotics, computer vision, speech recognition, and more.
So What is AI?
AI is a technology that enables computers and machines to simulate human intelligence. It's classified as weak and strong, with weak AI focusing on performing specific tasks, such as Apple's Siri and Amazon's Alexa, and strong AI, referring to AGI. Some experts suggest using the term augmented intelligence instead of "AI" to describe the weak AI used today and using "AI" only when referring to AGI. But there are still many other related terms, and mixing them up is easy.
Confusing Terminology
The jargon associated with artificial intelligence can be frustrating. Since AI products are released by anyone who feels like it, it's crucial to understand the terms to differentiate between valuable and useless information.
Here we go:
Machine Learning (ML) is a branch of AI that teaches machines to recognize data and get better at performing tasks on their own. Machines discover learning by looking at patterns and deciding what goes together and what goes next.
Deep Learning (DL) is a subset of ML; it uses multi-layered neural networks to mimic the complex decision-making power of the human brain.
Neural Network is an ML program or model that makes decisions like a human brain. It imitates the biological neural pathways of the brain by weighing options and drawing conclusions. There are three types of Neural Networks:
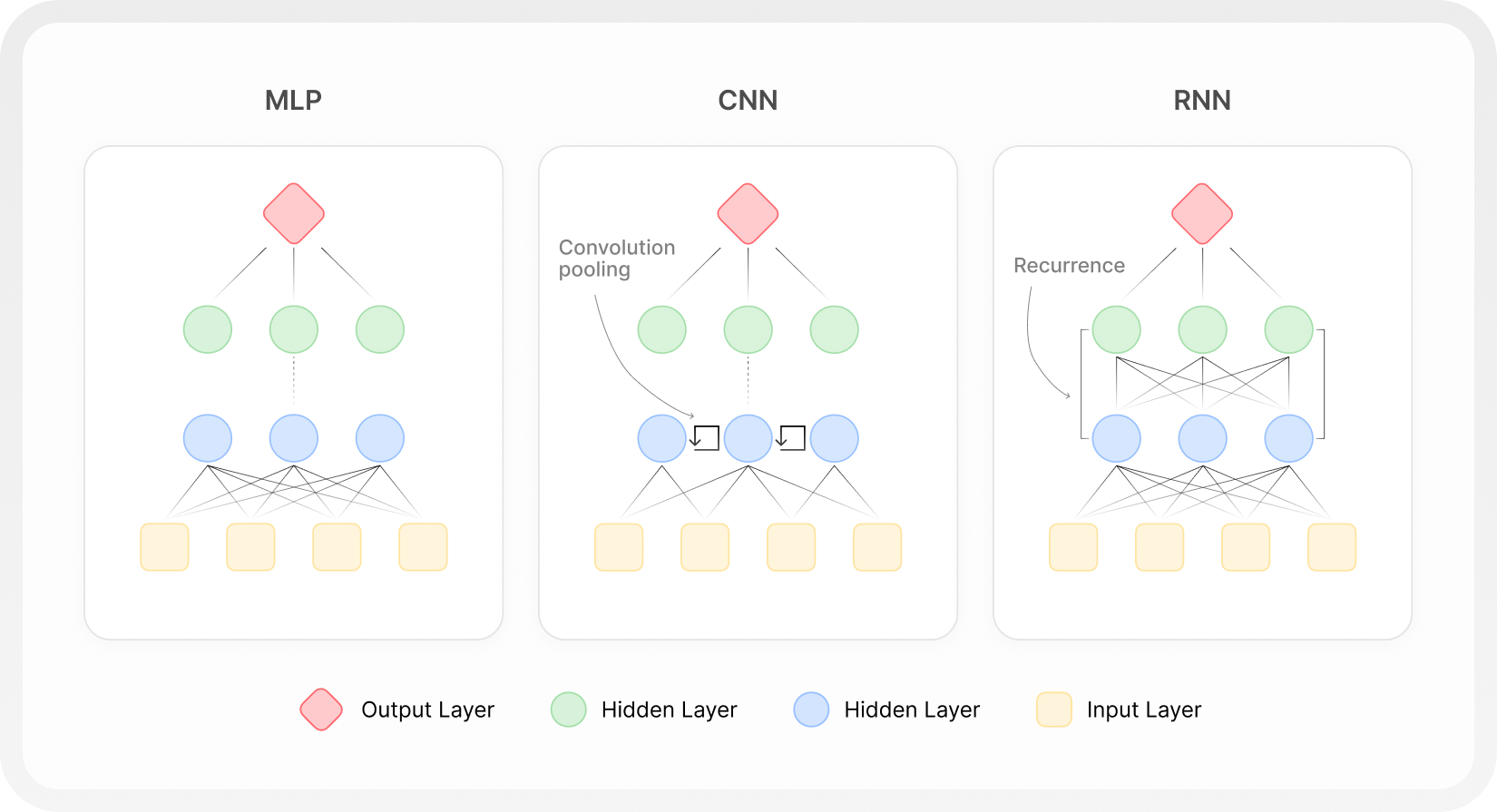
- Multi-Layer Perceptrons (MLP) are the most basic deep neural network, composed of fully connected layers. They're the basis of natural language processing and computer vision.
- Convolutional Neural Networks (CNN) are commonly used in computer vision. Given a series of images or videos from the real world, CNN learns to automatically extract the features of these inputs to complete specific tasks.
- Recurrent Neural Networks (RNN) are designed to handle sequential data and were specifically developed to predict future outcomes, like sales forecasting.
Natural Language Processing (NLP) is a field related to the interactions between computers and human languages. It's basically about how to program systems so they can understand natural language and extract relevant information from it.
Generative AI comprises DL models that can take raw data as input, "learn" from it, and generate probable outputs when prompted. ChatGPT is a prime example of generative AI.
Computer Vision is a field of AI that uses ML and neural networks to train systems to decode information from digital images, videos, and other visual inputs and make recommendations or take action when they see defects or issues.
Robotics focuses on the design and manufacturing of robots. Robots are made of electromechanical components that can exhibit or resemble human-level dexterity. They're also employed in fields where it can be challenging for humans to operate, such as on the assembly lines of car manufacturers or in medical surgery.
AI has revolutionary power in numerous fields, including medicine. For example, it helps diagnose patients and deliver outcomes at scale, assisting millions of doctors, especially in areas where primary care might not be readily available.
We've written before about how AI is being used in healthcare. AI-based technologies will undoubtedly continue to improve medicine; however, people are still susceptible to them. So, we decided to debunk the four most popular myths about AI in the medical field to illustrate that it's not as scary as it seems.
Myth #1: AI Will Replace Humans
AI's ability to take over humanity is often overstated due to inaccurate representations in media. However, AI is there to supplement and not replace knowledge workers.
A 2020 analysis of 200 social media posts took answers to a survey of whether AI would replace doctors. The results were 80% in favor of AI replacing human doctors, owing to more accurate diagnostics and access to a broader range of data. 20% of respondents, however, believe that AI can't replace human doctors, attributing to empathy, which they felt can't be accurately reflected by AI. In fact, artificial intelligence is a helpful guide and only implements part of the job.
Myth #2: AI Will Harm Patients
No, it won't. AI/ML-enabled devices are subject to the same FDA regulatory pathways as all other medical devices. The FDA oversees and carefully assesses the safety and effectiveness of AI/ML algorithms, focusing on data quality, robustness, and clinical performance. Furthermore, companies need to get FDA approval for any algorithm changes.
Myth #3: AI Can Do What Doctors Do
Not really. AI systems are designed to help doctors keep patients healthy and determine when they're not. However, AI can't choose the proper treatment because it doesn't understand cultural, family background, and economic status. It can predict cancer from images or find fractures in X-rays, but it can't give care or treatment alone.
Myth #4: Better Results with More Data
This thinking is a common misconception. More data doesn't necessarily translate to better results; data issues with quality and usability still remain. To draw safe, reliable assumptions, you need high-quality approaches that fix the messiness in the data. Without sufficient and well-represented data, you can't deliver on the AI promise in healthcare. Many steps must be taken, including digitalizing medical records and standardizing data infrastructure. Quality checks and patient confidentiality can be improved to enhance the quality of the collected data.
Final Thoughts
Companies advertise their products, claiming their creations are breakthroughs because of AI, but many contain algorithms and have nothing to do with artificial superintelligence. Unaware of the differences, people easily get hooked and eagerly pay for what they think is the almighty AI. Let's clear things up:
An algorithm is like a set of instructions that executes autonomously. Usually defined with some criteria, it processes incoming data based on those criteria. For example, YouTube suggests what videos a user should watch next.
Conversely, AI is a set of algorithms that can process unknown data but arrive at a predictable output format. It uses many ML algorithms to learn from previous outcomes and reach conclusions without human intervention.
Now that you understand the specifics of AI and can differentiate its various components, you're well-equipped to make more informed decisions.